Development and internal validation of a depression severity prediction model for tinnitus patients based on questionnaire responses and socio-demographics
Study predicting depression severity in tinnitus patients after treatment based on self-report questionnaire data acquired at baseline and ranking of features w.r.t. their attribution towards model prediction.
Paper authors: Uli Niemann, Petra Brueggemann, Benjamin Boecking, Birgit Mazurek, and Myra Spiliopoulou
Scientific Reports
By Uli Niemann in Research Tinnitus
March 13, 2020
Abstract
Tinnitus is a complex condition that is associated with major psychological and economic impairments - partly through various comorbidities such as depression. Understanding the interaction between tinnitus and depression may thus improve either symptom cluster’s prevention, diagnosis and treatment. In this study, we developed and validated a machine learning model to predict depression severity after outpatient therapy (T1) based on variables obtained before therapy (T0). 1,490 patients with chronic tinnitus (comorbid major depressive disorder: 52.2%) who completed a 7-day multimodal treatment encompassing tinnitus-specific components, cognitive behavioural therapy, physiotherapy and informational counselling were included. 185 variables were extracted from self-report questionnaires and socio-demographic data acquired at T0. We used 11 classification methods to train models that reliably separate between subclinical and clinical depression at T1 as measured by the general depression questionnaire. To ensure highly predictive and robust classifiers, we tuned algorithm hyperparameters in a 10-fold cross-validation scheme. To reduce model complexity and improve interpretability, we wrapped model training around an incremental feature selection mechanism that retained features that contributed to model prediction. We identified a LASSO model that included all 185 features to yield highest predictive performance (AUC = 0.87 ± 0.04). Through our feature selection wrapper, we identified a LASSO model with good trade-off between predictive performance and interpretability that used only 6 features (AUC = 0.85 ± 0.05). Thus, predictive machine learning models can lead to a better understanding of depression in tinnitus patients, and contribute to the selection of suitable therapeutic strategies and concise and valid questionnaire design for patients with chronic tinnitus with or without comorbid major depressive disorder.
Important figure
Figure 4. Predictive features. Distribution of features included in the lasso model of iteration i=7 for the patients with subclinical and clinical depression. Green squares and labels depict mean of continuous features.
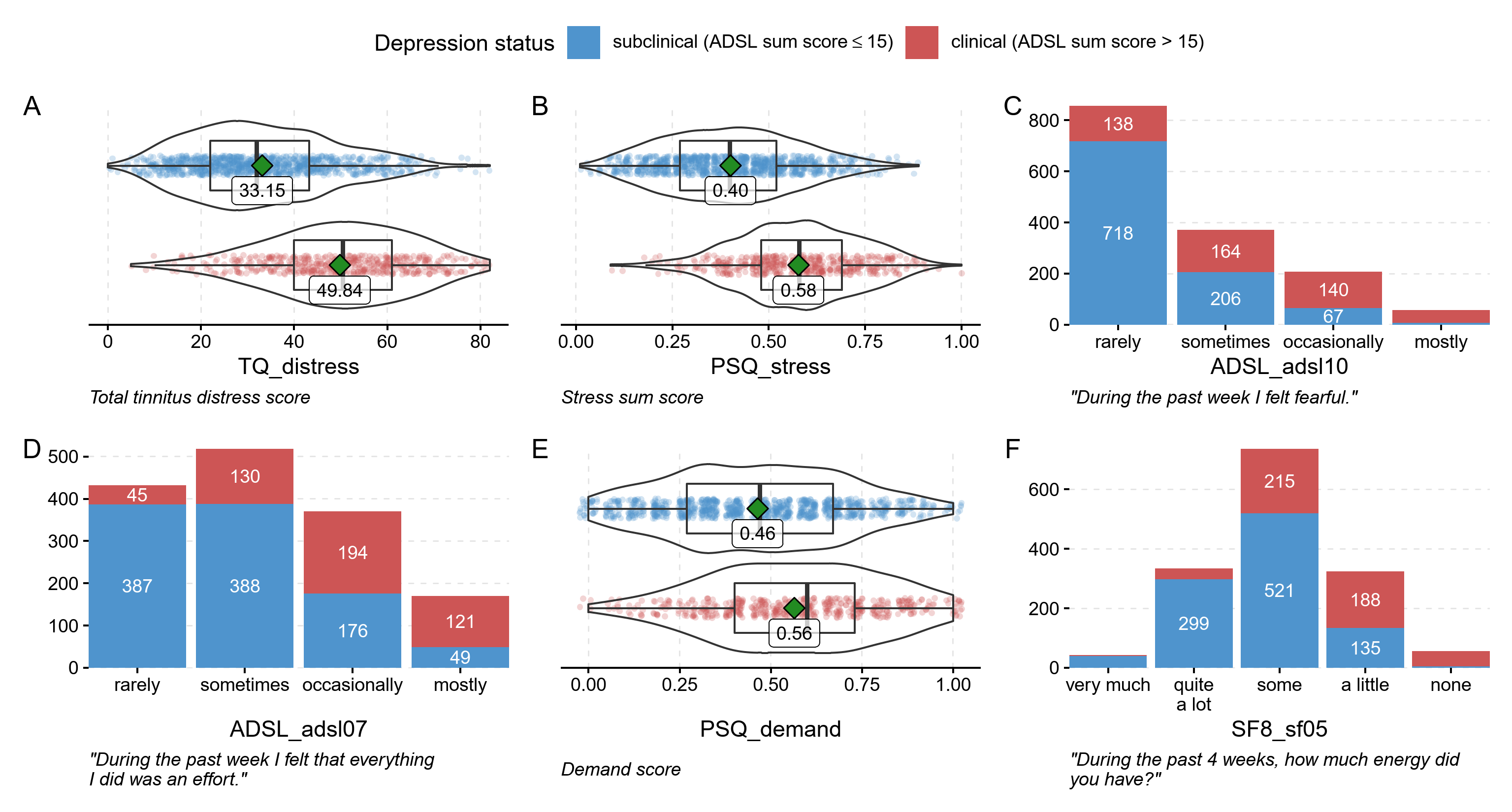
BibTeX citation
@article{Niemann:SREP2020,
author = {Niemann, Uli and Brueggemann, Petra and Boecking, Benjamin and
Mazurek, Birgit and Spiliopoulou, Myra},
journal = {Scientific Reports},
title = {Development and internal validation of a depression severity
prediction model for tinnitus patients based on questionnaire
responses and socio-demographics},
year = {2020},
issn = {2045-2322},
number = {4664},
pages = {1--9},
volume = {10},
doi = {10.1038/s41598-020-61593-z},
url = {https://doi.org/10.1038/s41598-020-61593-z},
}
- Posted on:
- March 13, 2020
- Length:
- 2 minute read, 398 words
- See Also:
- Data-Driven Prediction of Athletes’ Performance based on their Social Media Presence
- Classification of cardiac cohorts based on morphological and hemodynamic features derived from 4D PC-MRI data
- Gender-Specific Differences in Patients With Chronic Tinnitus - Baseline Characteristics and Treatment Effects